RISE West 2024: Fathom panel on autonomous risk-adjustment
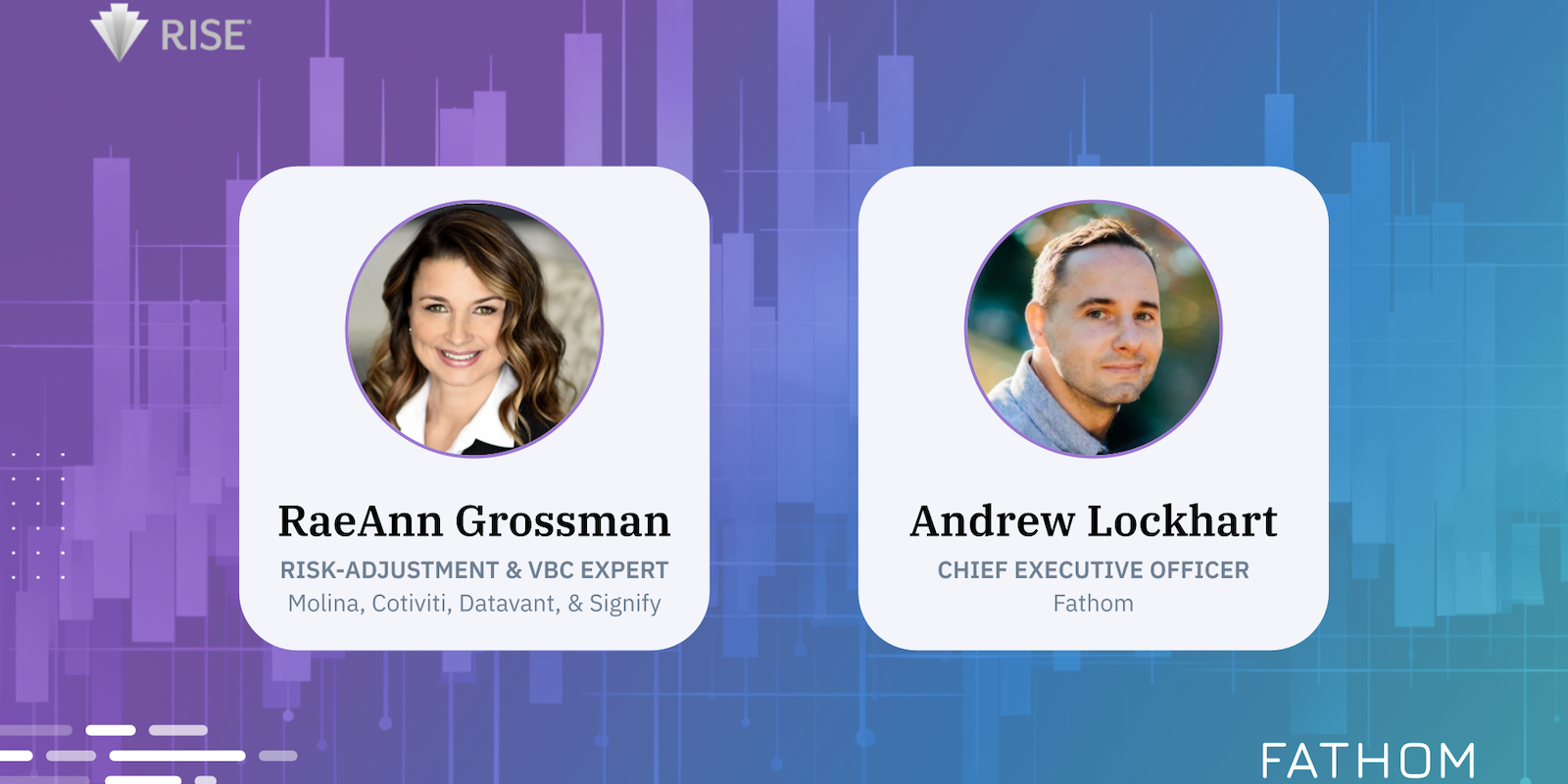
At RISE West in Colorado Springs (Sept. 11-13, 2024), industry experts RaeAnn Grossman – formerly of Molina, Cotiviti, Datavant, and Signify – and Andrew Lockhart, CEO of Fathom, discussed the evolution of technology for risk-adjustment coding to meet health plan and provider needs. This recap synthesizes key takeaways and actionable insights from the panel.
Key takeaways
1. Market and regulatory conditions are making risk-adjustment more challenging than ever, increasing the need for innovative solutions.
2. AI coding technology has matured significantly and now offers a clear solution tomany long-standing pain points for health plans and risk-based providers.
3. Early adopters of AI in risk-adjustment are gaining advantages in terms of cost,accuracy, and scalability of their coding operations.
Summary
1. Industry and regulatory update
Increasing demand strains resources. An aging population and an increasing shift to value-based care are driving both a higher volume and a higher complexity of comprehensive health status overviews, putting pressure on coding operations to do more with less.
Vendor landscape is in flux. Consolidation has reduced options, while an influx of startups has made vendor selection more complex. Health plans must ask pointed discovery questions to navigate this changing vendor landscape and find needed supply.
Regulatory scrutiny is intensifying. With OIG releasing new audit toolkits, plans face heightened compliance pressures. This includes the requirement for three certified coders to agree on HCC presence during audits, further increasing costs on audits and reviews.
Dual coding systems add complexity. The transition from v24 to v28 models increases the number of HCCs while reducing mappable ICDs, necessitating additional training and validation efforts. Under manual coding approaches, this retraining can take weeks or months for risk-adjustment coders to attain proficiency.
Drive for completeness and accuracy. Organizations need to extract complete detail from each chart review, obtaining both risk-adjustment and quality measures in fewer passes. Capturing every supported HCC and determining RAF scores with greater than 95% accuracy is paramount – not just for audit compliance, but to ensure appropriate care and treatment.
Leaders prioritize workforce development, scalability, and velocity. As the industry adopts AI, organizations must invest in developing their teams' capabilities to evaluate, deploy, and manage AI effectively. This underscores a shift from "managing people" to "managing software." With the next generation of technology in place, leaders can scale their coding operations sustainably and increase velocity without compromising quality or compliance.
2. Technology evolution
Rules-based NLP marked the early days. Natural language processing (NLP) tools of the 2000s to mid-2010s offered limited automation for simple scenarios, but struggled with risk-adjustment due to chart length and complexity.
ICD-10 implementation created new challenges. The 2015 transition to ICD-10 increased the number of codes tenfold, multiplying the ways clinicians could express diagnoses. This dramatic increase in complexity caused existing automation technology to falter.
Deep learning emerged as a game-changer. Around 2018 onward, deep learning AI enabled end-to-end automation of medical coding. Each year has seen improvements in coverage, automation rates, and accuracy across specialties. In 2021, deep learning started powering risk-adjustment tools for both health plans and providers and hitting accuracy benchmarks.
3. Case study
Early adopter achieves >90% automation. A health plan with 2 million members, including 400,000+ on Medicare Advantage, implemented deep-learning AI with impressive results.
Dramatic reduction in manual review. With 91.2% of charts fully automated, the internal team could focus on the remaining complex cases while improving security and compliance.
Improved accuracy drives better outcomes. A 38% reduction in coding errors led to a 27% increase in ICD capture, resulting in more accurate reimbursement and reduced audit risk. As noted, "The machine doesn't value its time, so it looks at every page. It doesn't have to work faster to hit productivity benchmarks."
Significant cost savings realized. The organization saw a 47.8% reduction in vendor coding spend, freeing up resources for other strategic initiatives.
Faster processing enhances financial performance. Cloud-based AI allowed all charts to be coded within 24 hours, regardless of volume, improving cash flow and revenue visibility.
4. Implications
Rigorous vendor evaluation is critical. To vet AI vendors, conduct thorough discovery processes and test the technology at scale. Running the AI on substantive volume – for example, 10,000+ charts – and auditing the results will demonstrate its capabilities and ensure that the vendor is truly using technology, not a tech-enabled service.
Consider team strength alongside technology. The right partner brings more than just technology. Look beyond the software to evaluate the vendor's team: Seek partners with top-notch project managers (e.g., former BCG/McKinsey consultants) to truly understand your unique context, set clear objectives, guide implementation, manage stakeholders, and establish robust evaluation frameworks.
Compliance must be at the forefront. Involve compliance teams early in the vendor selection process. Evaluate how the AI documents its decision making and how the vendor assesses its own performance internally. This proactive approach can help mitigate audit risks and ensure the solution meets rigorous compliance standards.
If you'd like to learn more about Fathom, schedule a meeting here.
Related Posts
Stay up to date
Get the latest in industry news and insights delivered straight to your inbox.